在这篇文章中,我们将为您详细介绍Kafka+SparkStreaming+Redis实时计算整合实践的内容,并且讨论关于kafkaredis性能的相关问题。此外,我们还会涉及一些关于23友盟项目--s
在这篇文章中,我们将为您详细介绍Kafka+Spark Streaming+Redis实时计算整合实践的内容,并且讨论关于kafka redis性能的相关问题。此外,我们还会涉及一些关于23 友盟项目--sparkstreaming对接kafka、集成redis--从redis中查询月留存率、Flume+Kafka+SparkStreaming 最新最全整合、java8下spark-streaming结合kafka编程(spark 2.0 & kafka 0.10、kafka(08) + spark streaming(java) + redis 网站访问统计demo的知识,以帮助您更全面地了解这个主题。
本文目录一览:
Kafka+Spark Streaming+Redis实时计算整合实践(kafka redis性能)
基于Spark通用计算平台,可以很好地扩展各种计算类型的应用,尤其是Spark提供了内建的计算库支持,像Spark Streaming、Spark SQL、MLlib、GraphX,这些内建库都提供了高级抽象,可以用非常简洁的代码实现复杂的计算逻辑、这也得益于Scala编程语言的简洁性。这 里,我们基于1.3.0版本的Spark搭建了计算平台,实现基于Spark Streaming的实时计算。
我们的应用场景是分析用户使用手机App的行为,描述如下所示:
手机客户端会收集用户的行为事件(我们以点击事件为例),将数据发送到数据服务器,我们假设这里直接进入到Kafka消息队列
后端的实时服务会从Kafka消费数据,将数据读出来并进行实时分析,这里选择Spark Streaming,因为Spark Streaming提供了与Kafka整合的内置支持
经过Spark Streaming实时计算程序分析,将结果写入Redis,可以实时获取用户的行为数据,并可以导出进行离线综合统计分析
Spark Streaming介绍
Spark Streaming提供了一个叫做DStream(Discretized Stream)的高级抽象,DStream表示一个持续不断输入的数据流,可以基于Kafka、TCP Socket、Flume等输入数据流创建。在内部,一个DStream实际上是由一个RDD序列组成的。Sparking Streaming是基于Spark平台的,也就继承了Spark平台的各种特性,如容错(Fault-tolerant)、可扩展 (Scalable)、高吞吐(High-throughput)等。
在Spark Streaming中,每个DStream包含了一个时间间隔之内的数据项的集合,我们可以理解为指定时间间隔之内的一个batch,每一个batch就 构成一个RDD数据集,所以DStream就是一个个batch的有序序列,时间是连续的,按照时间间隔将数据流分割成一个个离散的RDD数据集,如图所 示(来自官网):
我们都知道,Spark支持两种类型操作:Transformations和Actions。Transformation从一个已知的RDD数据集经过 转换得到一个新的RDD数据集,这些Transformation操作包括map、filter、flatMap、union、join等,而且 Transformation具有lazy的特性,调用这些操作并没有立刻执行对已知RDD数据集的计算操作,而是在调用了另一类型的Action操作才 会真正地执行。Action执行,会真正地对RDD数据集进行操作,返回一个计算结果给Driver程序,或者没有返回结果,如将计算结果数据进行持久 化,Action操作包括reduceByKey、count、foreach、collect等。关于Transformations和Actions 更详细内容,可以查看官网文档。
同样、Spark Streaming提供了类似Spark的两种操作类型,分别为Transformations和Output操作,它们的操作对象是DStream,作 用也和Spark类似:Transformation从一个已知的DStream经过转换得到一个新的DStream,而且Spark Streaming还额外增加了一类针对Window的操作,当然它也是Transformation,但是可以更灵活地控制DStream的大小(时间 间隔大小、数据元素个数),例如window(windowLength, slideInterval)、countByWindow(windowLength, slideInterval)、reduceByWindow(func, windowLength, slideInterval)等。Spark Streaming的Output操作允许我们将DStream数据输出到一个外部的存储系统,如数据库或文件系统等,执行Output操作类似执行 Spark的Action操作,使得该操作之前lazy的Transformation操作序列真正地执行。
Kafka+Spark Streaming+Redis编程实践
下面,我们根据上面提到的应用场景,来编程实现这个实时计算应用。首先,写了一个Kafka Producer模拟程序,用来模拟向Kafka实时写入用户行为的事件数据,数据是JSON格式,示例如下:
1 |
{"uid":"068b746ed4620d25e26055a9f804385f","event_time":"1430204612405","os_type":"Android","click_count":6} |
一个事件包含4个字段:
uid:用户编号
event_time:事件发生时间戳
os_type:手机App操作系统类型
click_count:点击次数
下面是我们实现的代码,如下所示:
01 |
package org.shirdrn.spark.streaming.utils |
03 |
import java.util.Properties |
04 |
import scala.util.Properties |
05 |
import org.codehaus.jettison.json.JSONObject |
06 |
import kafka.javaapi.producer.Producer |
07 |
import kafka.producer.KeyedMessage |
08 |
import kafka.producer.KeyedMessage |
09 |
import kafka.producer.ProducerConfig |
10 |
import scala.util.Random |
12 |
object KafkaEventProducer { |
14 |
private val users = Array( |
15 |
"4A4D769EB9679C054DE81B973ED5D768" , "8dfeb5aaafc027d89349ac9a20b3930f" , |
16 |
"011BBF43B89BFBF266C865DF0397AA71" , "f2a8474bf7bd94f0aabbd4cdd2c06dcf" , |
17 |
"068b746ed4620d25e26055a9f804385f" , "97edfc08311c70143401745a03a50706" , |
18 |
"d7f141563005d1b5d0d3dd30138f3f62" , "c8ee90aade1671a21336c721512b817a" , |
19 |
"6b67c8c700427dee7552f81f3228c927" , "a95f22eabc4fd4b580c011a3161a9d9d" ) |
21 |
private val random = new Random() |
23 |
private var pointer = - 1 |
25 |
def getUserID() : String = { |
27 |
if (pointer > = users.length) { |
35 |
def click() : Double = { |
39 |
// bin/kafka-topics.sh --zookeeper zk1:2181,zk2:2181,zk3:2181/kafka --create --topic user_events --replication-factor 2 --partitions 2 |
40 |
// bin/kafka-topics.sh --zookeeper zk1:2181,zk2:2181,zk3:2181/kafka --list |
41 |
// bin/kafka-topics.sh --zookeeper zk1:2181,zk2:2181,zk3:2181/kafka --describe user_events |
42 |
// bin/kafka-console-consumer.sh --zookeeper zk1:2181,zk2:2181,zk3:22181/kafka --topic test_json_basis_event --from-beginning |
43 |
def main(args : Array[String]) : Unit = { |
44 |
val topic = "user_events" |
45 |
val brokers = "10.10.4.126:9092,10.10.4.127:9092" |
46 |
val props = new Properties() |
47 |
props.put( "metadata.broker.list" , brokers) |
48 |
props.put( "serializer.class" , "kafka.serializer.StringEncoder" ) |
50 |
val kafkaConfig = new ProducerConfig(props) |
51 |
val producer = new Producer[String, String](kafkaConfig) |
55 |
val event = new JSONObject() |
57 |
.put( "uid" , getUserID) |
58 |
.put( "event_time" , System.currentTimeMillis.toString) |
59 |
.put( "os_type" , "Android" ) |
60 |
.put( "click_count" , click) |
62 |
// produce event message |
63 |
producer.send( new KeyedMessage[String, String](topic, event.toString)) |
64 |
println( "Message sent: " + event) |
通过控制上面程序最后一行的时间间隔来控制模拟写入速度。下面我们来讨论实现实时统计每个用户的点击次数,它是按照用户分组进行累加次数,逻辑比较简单,关键是在实现过程中要注意一些问题,如对象序列化等。先看实现代码,稍后我们再详细讨论,代码实现如下所示:
01 |
object UserClickCountAnalytics { |
03 |
def main(args : Array[String]) : Unit = { |
04 |
var masterUrl = "local[1]" |
05 |
if (args.length > 0 ) { |
09 |
// Create a StreamingContext with the given master URL |
10 |
val conf = new SparkConf().setMaster(masterUrl).setAppName( "UserClickCountStat" ) |
11 |
val ssc = new StreamingContext(conf, Seconds( 5 )) |
13 |
// Kafka configurations |
14 |
val topics = Set( "user_events" ) |
15 |
val brokers = "10.10.4.126:9092,10.10.4.127:9092" |
16 |
val kafkaParams = Map[String, String]( |
17 |
"metadata.broker.list" -> brokers, "serializer.class" -> "kafka.serializer.StringEncoder" ) |
20 |
val clickHashKey = "app::users::click" |
22 |
// Create a direct stream |
23 |
val kafkaStream = KafkaUtils.createDirectStream[String, String, StringDecoder, StringDecoder](ssc, kafkaParams, topics) |
25 |
val events = kafkaStream.flatMap(line = > { |
26 |
val data = JSONObject.fromObject(line. _ 2 ) |
30 |
// Compute user click times |
31 |
val userClicks = events.map(x = > (x.getString( "uid" ), x.getInt( "click_count" ))).reduceByKey( _ + _ ) |
32 |
userClicks.foreachRDD(rdd = > { |
33 |
rdd.foreachPartition(partitionOfRecords = > { |
34 |
partitionOfRecords.foreach(pair = > { |
36 |
val clickCount = pair. _ 2 |
37 |
val jedis = RedisClient.pool.getResource |
39 |
jedis.hincrBy(clickHashKey, uid, clickCount) |
40 |
RedisClient.pool.returnResource(jedis) |
46 |
ssc.awaitTermination() |
上面代码使用了Jedis客户端来操作Redis,将分组计数结果数据累加写入Redis存储,如果其他系统需要实时获取该数据,直接从Redis实时读取即可。RedisClient实现代码如下所示:
01 |
object RedisClient extends Serializable { |
02 |
val redisHost = "10.10.4.130" |
04 |
val redisTimeout = 30000 |
05 |
lazy val pool = new JedisPool( new GenericObjectPoolConfig(), redisHost, redisPort, redisTimeout) |
07 |
lazy val hook = new Thread { |
09 |
println( "Execute hook thread: " + this ) |
13 |
sys.addShutdownHook(hook.run) |
上面代码我们分别在local[K]和Spark Standalone集群模式下运行通过。
如果我们是在开发环境进行调试的时候,也就是使用local[K]部署模式,在本地启动K个Worker线程来计算,这K个Worker在同一个JVM实 例里,上面的代码默认情况是,如果没有传参数则是local[K]模式,所以如果使用这种方式在创建Redis连接池或连接的时候,可能非常容易调试通 过,但是在使用Spark Standalone、YARN Client(YARN Cluster)或Mesos集群部署模式的时候,就会报错,主要是由于在处理Redis连接池或连接的时候出错了。我们可以看一下Spark架构,如图 所示(来自官网):
无论是在本地模式、Standalone模式,还是在Mesos或YARN模式下,整个Spark集群的结构都可以用上图抽象表示,只是各个组件的运行环 境不同,导致组件可能是分布式的,或本地的,或单个JVM实例的。如在本地模式,则上图表现为在同一节点上的单个进程之内的多个组件;而在YARN Client模式下,Driver程序是在YARN集群之外的一个节点上提交Spark Application,其他的组件都运行在YARN集群管理的节点上。
在Spark集群环境部署Application后,在进行计算的时候会将作用于RDD数据集上的函数(Functions)发送到集群中Worker上 的Executor上(在Spark Streaming中是作用于DStream的操作),那么这些函数操作所作用的对象(Elements)必须是可序列化的,通过Scala也可以使用 lazy引用来解决,否则这些对象(Elements)在跨节点序列化传输后,无法正确地执行反序列化重构成实际可用的对象。上面代码我们使用lazy引 用(Lazy Reference)来实现的,代码如下所示:
01 |
// lazy pool reference |
02 |
lazy val pool = new JedisPool( new GenericObjectPoolConfig(), redisHost, redisPort, redisTimeout) |
04 |
partitionOfRecords.foreach(pair = > { |
06 |
val clickCount = pair. _ 2 |
07 |
val jedis = RedisClient.pool.getResource |
09 |
jedis.hincrBy(clickHashKey, uid, clickCount) |
10 |
RedisClient.pool.returnResource(jedis) |
另一种方式,我们将代码修改为,把对Redis连接的管理放在操作DStream的Output操作范围之内,因为我们知道它是在特定的Executor中进行初始化的,使用一个单例的对象来管理,如下所示:
001 |
package org.shirdrn.spark.streaming |
003 |
import org.apache.commons.pool 2 .impl.GenericObjectPoolConfig |
004 |
import org.apache.spark.SparkConf |
005 |
import org.apache.spark.streaming.Seconds |
006 |
import org.apache.spark.streaming.StreamingContext |
007 |
import org.apache.spark.streaming.dstream.DStream.toPairDStreamFunctions |
008 |
import org.apache.spark.streaming.kafka.KafkaUtils |
010 |
import kafka.serializer.StringDecoder |
011 |
import net.sf.json.JSONObject |
012 |
import redis.clients.jedis.JedisPool |
014 |
object UserClickCountAnalytics { |
016 |
def main(args : Array[String]) : Unit = { |
017 |
var masterUrl = "local[1]" |
018 |
if (args.length > 0 ) { |
022 |
// Create a StreamingContext with the given master URL |
023 |
val conf = new SparkConf().setMaster(masterUrl).setAppName( "UserClickCountStat" ) |
024 |
val ssc = new StreamingContext(conf, Seconds( 5 )) |
026 |
// Kafka configurations |
027 |
val topics = Set( "user_events" ) |
028 |
val brokers = "10.10.4.126:9092,10.10.4.127:9092" |
029 |
val kafkaParams = Map[String, String]( |
030 |
"metadata.broker.list" -> brokers, "serializer.class" -> "kafka.serializer.StringEncoder" ) |
033 |
val clickHashKey = "app::users::click" |
035 |
// Create a direct stream |
036 |
val kafkaStream = KafkaUtils.createDirectStream[String, String, StringDecoder, StringDecoder](ssc, kafkaParams, topics) |
038 |
val events = kafkaStream.flatMap(line = > { |
039 |
val data = JSONObject.fromObject(line. _ 2 ) |
043 |
// Compute user click times |
044 |
val userClicks = events.map(x = > (x.getString( "uid" ), x.getInt( "click_count" ))).reduceByKey( _ + _ ) |
045 |
userClicks.foreachRDD(rdd = > { |
046 |
rdd.foreachPartition(partitionOfRecords = > { |
047 |
partitionOfRecords.foreach(pair = > { |
050 |
* Internal Redis client for managing Redis connection {@link Jedis} based on {@link RedisPool} |
052 |
object InternalRedisClient extends Serializable { |
054 |
@ transient private var pool : JedisPool = null |
056 |
def makePool(redisHost : String, redisPort : Int, redisTimeout : Int, |
057 |
maxTotal : Int, maxIdle : Int, minIdle : Int) : Unit = { |
058 |
makePool(redisHost, redisPort, redisTimeout, maxTotal, maxIdle, minIdle, true , false , 10000 ) |
061 |
def makePool(redisHost : String, redisPort : Int, redisTimeout : Int, |
062 |
maxTotal : Int, maxIdle : Int, minIdle : Int, testOnBorrow : Boolean, |
063 |
testOnReturn : Boolean, maxWaitMillis : Long) : Unit = { |
065 |
val poolConfig = new GenericObjectPoolConfig() |
066 |
poolConfig.setMaxTotal(maxTotal) |
067 |
poolConfig.setMaxIdle(maxIdle) |
068 |
poolConfig.setMinIdle(minIdle) |
069 |
poolConfig.setTestOnBorrow(testOnBorrow) |
070 |
poolConfig.setTestOnReturn(testOnReturn) |
071 |
poolConfig.setMaxWaitMillis(maxWaitMillis) |
072 |
pool = new JedisPool(poolConfig, redisHost, redisPort, redisTimeout) |
074 |
val hook = new Thread{ |
075 |
override def run = pool.destroy() |
077 |
sys.addShutdownHook(hook.run) |
081 |
def getPool : JedisPool = { |
087 |
// Redis configurations |
091 |
val redisHost = "10.10.4.130" |
093 |
val redisTimeout = 30000 |
095 |
InternalRedisClient.makePool(redisHost, redisPort, redisTimeout, maxTotal, maxIdle, minIdle) |
098 |
val clickCount = pair. _ 2 |
099 |
val jedis = InternalRedisClient.getPool.getResource |
100 |
jedis.select(dbIndex) |
101 |
jedis.hincrBy(clickHashKey, uid, clickCount) |
102 |
InternalRedisClient.getPool.returnResource(jedis) |
108 |
ssc.awaitTermination() |
上面代码实现,得益于Scala语言的特性,可以在代码中任何位置进行class或object的定义,我们将用来管理Redis连接的代码放在了 特定操作的内部,就避免了瞬态(Transient)对象跨节点序列化的问题。这样做还要求我们能够了解Spark内部是如何操作RDD数据集的,更多可 以参考RDD或Spark相关文档。
在集群上,以Standalone模式运行,执行如下命令:
2 |
./bin/spark-submit --class org.shirdrn.spark.streaming.UserClickCountAnalytics --master spark://hadoop1:7077 --executor-memory 1G --total-executor-cores 2 ~/spark-0.0.SNAPSHOT.jar spark://hadoop1:7077 |
可以查看集群中各个Worker节点执行计算任务的状态,也可以非常方便地通过Web页面查看。
下面,看一下我们存储到Redis中的计算结果,如下所示:
01 |
127.0.0.1:6379[1]> HGETALL app::users::click |
02 |
1) "4A4D769EB9679C054DE81B973ED5D768" |
04 |
3) "8dfeb5aaafc027d89349ac9a20b3930f" |
06 |
5) "011BBF43B89BFBF266C865DF0397AA71" |
08 |
7) "97edfc08311c70143401745a03a50706" |
10 |
9) "d7f141563005d1b5d0d3dd30138f3f62" |
12 |
11) "a95f22eabc4fd4b580c011a3161a9d9d" |
14 |
13) "6b67c8c700427dee7552f81f3228c927" |
16 |
15) "f2a8474bf7bd94f0aabbd4cdd2c06dcf" |
18 |
17) "c8ee90aade1671a21336c721512b817a" |
20 |
19) "068b746ed4620d25e26055a9f804385f" |
有关更多关于Spark Streaming的详细内容,可以参考官方文档。
附录
这里,附上前面开发的应用所对应的依赖,以及打包Spark Streaming应用程序的Maven配置,以供参考。如果使用maven-shade-plugin插件,配置有问题的话,打包后在Spark集群上 提交Application时候可能会报错Invalid signature file digest for Manifest main attributes。参考的Maven配置,如下所示:
001 |
< project xmlns = "http://maven.apache.org/POM/4.0.0" xmlns:xsi = "http://www.w3.org/2001/XMLSchema-instance" |
002 |
xsi:schemaLocation = "http://maven.apache.org/POM/4.0.0 http://maven.apache.org/xsd/maven-4.0.0.xsd" > |
003 |
< modelVersion >4.0.0</ modelVersion > |
004 |
< groupId >org.shirdrn.spark</ groupId > |
005 |
< artifactId >spark</ artifactId > |
006 |
< version >0.0.1-SNAPSHOT</ version > |
010 |
< groupId >org.apache.spark</ groupId > |
011 |
< artifactId >spark-core_2.10</ artifactId > |
012 |
< version >1.3.0</ version > |
015 |
< groupId >org.apache.spark</ groupId > |
016 |
< artifactId >spark-streaming_2.10</ artifactId > |
017 |
< version >1.3.0</ version > |
020 |
< groupId >net.sf.json-lib</ groupId > |
021 |
< artifactId >json-lib</ artifactId > |
022 |
< version >2.3</ version > |
025 |
< groupId >org.apache.spark</ groupId > |
026 |
< artifactId >spark-streaming-kafka_2.10</ artifactId > |
027 |
< version >1.3.0</ version > |
030 |
< groupId >redis.clients</ groupId > |
031 |
< artifactId >jedis</ artifactId > |
032 |
< version >2.5.2</ version > |
035 |
< groupId >org.apache.commons</ groupId > |
036 |
< artifactId >commons-pool2</ artifactId > |
037 |
< version >2.2</ version > |
042 |
< sourceDirectory >${basedir}/src/main/scala</ sourceDirectory > |
043 |
< testSourceDirectory >${basedir}/src/test/scala</ testSourceDirectory > |
046 |
< directory >${basedir}/src/main/resources</ directory > |
051 |
< directory >${basedir}/src/test/resources</ directory > |
056 |
< artifactId >maven-compiler-plugin</ artifactId > |
057 |
< version >3.1</ version > |
064 |
< groupId >org.apache.maven.plugins</ groupId > |
065 |
< artifactId >maven-shade-plugin</ artifactId > |
066 |
< version >2.2</ version > |
068 |
< createDependencyReducedPom >true</ createDependencyReducedPom > |
072 |
< phase >package</ phase > |
079 |
< include >*:*</ include > |
084 |
< artifact >*:*</ artifact > |
086 |
< exclude >META-INF/*.SF</ exclude > |
087 |
< exclude >META-INF/*.DSA</ exclude > |
088 |
< exclude >META-INF/*.RSA</ exclude > |
094 |
implementation = "org.apache.maven.plugins.shade.resource.ServicesResourceTransformer" /> |
096 |
implementation = "org.apache.maven.plugins.shade.resource.AppendingTransformer" > |
097 |
< resource >reference.conf</ resource > |
100 |
implementation = "org.apache.maven.plugins.shade.resource.DontIncludeResourceTransformer" > |
101 |
< resource >log4j.properties</ resource > |
参考链接
http://spark.apache.org/docs/1.3.0/index.html
http://spark.apache.org/docs/1.3.0/cluster-overview.html
http://spark.apache.org/docs/1.3.0/job-scheduling.html
http://spark.apache.org/docs/1.3.0/streaming-programming-guide.html
http://stackoverflow.com/questions/28006517/redis-on-sparktask-not-serializable

本文基于署名-非商业性使用-相同方式共享 4.0许可协议发布,欢迎转载、使用、重新发布,但务必保留文章署名时延军(包含链接:http://shiyanjun.cn),不得用于商业目的,基于本文修改后的作品务必以相同的许可发布。如有任何疑问,请与我联系。
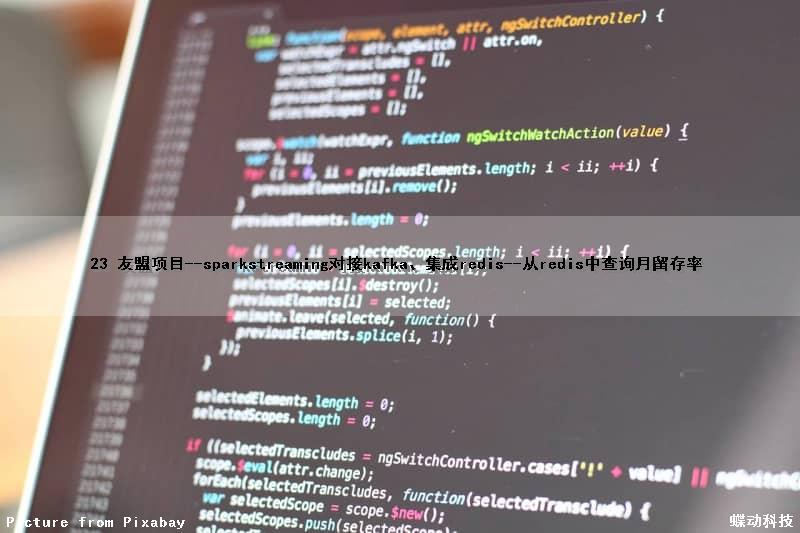
23 友盟项目--sparkstreaming对接kafka、集成redis--从redis中查询月留存率
从redis中查询月留存率
StatRemainRatioMonth
1 package com.oldboy.umeng.spark.stat;
2
3
4
5 /**
6 * 统计月留存率
7 */
8 public class StatRemainRatioMonth {
9 public static void main(String[] args) throws Exception {
10 SparkConf conf = new SparkConf();
11 conf.setAppName("statNew");
12 conf.setMaster("local[4]");
13 SparkSession sess = SparkSession.builder().config(conf).enableHiveSupport().getOrCreate();
14
15 //sess.sql("select * from row_log");
16 //注册函数
17 ExecSQLUtil.execRegisterFuncs(sess);
18
19 String day = "20181105" ;
20 Jedis redis = new Jedis("s101" , 6379) ;
21 redis.select(1) ;
22 Set<String> keys = redis.keys("*") ;
23 //创建Row行类型List集合
24 List<Row> rows = new ArrayList<Row>() ;
25 for(String key :keys){
26 String[] arr = key.split("#") ;
27 String value = redis.get(key) ;
28 long mintime = Long.parseLong(value.split(",")[0]) ;//redis中取出最小值
29 System.out.println(DateUtil.formatDay(mintime , 0 , "yyyyMMdd"));
30 rows.add(RowFactory.create(arr[0], arr[1], arr[2], arr[3], arr[4], arr[5], arr[6],mintime)) ;
31 }
32 //创建java rdd
33 JavaRDD<Row> rdd1 = new JavaSparkContext(sess.sparkContext()).parallelize(rows);
34 //结构化字段
35 StructField[] fields = new StructField[8];
36 fields[0] = new StructField("appid", DataTypes.StringType, false, Metadata.empty());
37 fields[1] = new StructField("appversion", DataTypes.StringType, false, Metadata.empty());
38 fields[2] = new StructField("brand", DataTypes.StringType, false, Metadata.empty());
39 fields[3] = new StructField("appplatform", DataTypes.StringType, false, Metadata.empty());
40 fields[4] = new StructField("devicestyle", DataTypes.StringType, false, Metadata.empty());
41 fields[5] = new StructField("ostype", DataTypes.StringType, false, Metadata.empty());
42 fields[6] = new StructField("deviceid", DataTypes.StringType, false, Metadata.empty());
43 fields[7] = new StructField("mintime", DataTypes.LongType, false, Metadata.empty());
44 //指定schame类型
45 StructType type = new StructType(fields);
46 //创建Dataset 数据表
47 Dataset<Row> ds1 = sess.createDataFrame(rdd1 ,type ) ;
48 //数据表创建为临时表
49 ds1.createOrReplaceTempView("_tt");
50
51 //新增设备 各个维度下的 条件:最小值所在的月 = 给出查询的时间所在的月
52 String sql = "select appid ,appversion,brand,appplatform,devicestyle,ostype,deviceid " +
53 "from _tt " +
54 "where formatbymonth(mintime, 0 , ''yyyyMM'') = formatbymonth(''"+ day+"'' , ''yyyyMMdd'' , 0 , ''yyyyMM'')" +
55 "group by appid ,appversion,brand,appplatform,devicestyle,ostype,deviceid " +
56 "with cube" ;
57
58 sess.sql(sql).createOrReplaceTempView("_t2");//再创建一个临时表
59 System.out.println("========================");
60 //有效的新增设备,注册v1视图 //设备id 和app 不能为空 其他字段如果为null,转为NULL,因为null不参与统计
61 sess.sql("select ifnull(ss.appid ,''NULLL'') appid ," +
62 "ifnull(ss.appversion ,''NULLL'') appversion ," +
63 "ifnull(ss.appplatform ,''NULLL'') appplatform ," +
64 "ifnull(ss.brand ,''NULLL'') brand ," +
65 "ifnull(ss.devicestyle ,''NULLL'') devicestyle ," +
66 "ifnull(ss.ostype,''NULLL'') ostype ," +
67 "ifnull(ss.deviceid ,''NULLL'') deviceid " +
68 " from _t2 ss" +
69 " where ss.appid is not null and ss.deviceid is not null")
70 .createOrReplaceTempView("v1");
71 sess.sql("select * from v1").show(1000,false);
72 //
73 String sql2 = ResourceUtil.readResourceAsString("stat_remainratio_month2.sql") ;
74 sql2 = sql2.replace("${ymd}" , day) ;
75 //
76 //执行sql语句
77 ExecSQLUtil.execSQLString(sess , sql2);
78 }
79 }
执行sql语句
-- 计算留存率
use big12_umeng ;
-- 查询经过一个月后的活跃用户-
CREATE OR replace TEMPORARY view v2 as
SELECT
ifnull(s.appid ,''NULLL'') appid ,
ifnull(s.appversion ,''NULLL'') appversion ,
ifnull(s.appplatform ,''NULLL'') appplatform ,
ifnull(s.brand ,''NULLL'') brand ,
ifnull(s.devicestyle ,''NULLL'') devicestyle ,
ifnull(s.ostype ,''NULLL'') ostype ,
ifnull(s.deviceid ,''NULLL'') deviceid
FROM
(
SELECT
appid ,
appversion ,
appplatform ,
brand ,
devicestyle ,
ostype ,
deviceid
FROM
appstartuplogs
WHERE
ym = formatbymonth(''${ymd}'' , ''yyyyMMdd'' , 1 , ''yyyyMM'')
group by
appid ,
appversion ,
appplatform ,
brand ,
devicestyle ,
ostype ,
deviceid
with cube
)s
WHERE
s.appid is not NULL
and s.deviceid is not null ;
--
-- 查询交集()
CREATE OR replace TEMPORARY view v3 as
SELECT
v1.appid ,
v1.appversion ,
v1.appplatform ,
v1.brand ,
v1.devicestyle ,
v1.ostype ,
count(v1.deviceid) cnt
FROM
v1,v2
WHERE
v1.appid = v2.appid
and v1.appversion = v2.appversion
and v1.appplatform = v2.appplatform
and v1.brand = v2.brand
and v1.devicestyle = v2.devicestyle
and v1.ostype = v2.ostype
and v1.deviceid = v2.deviceid
GROUP BY
v1.appid ,
v1.appversion ,
v1.appplatform ,
v1.brand ,
v1.devicestyle ,
v1.ostype ;
CREATE OR replace TEMPORARY view v4 as
SELECT
v1.appid ,
v1.appversion ,
v1.appplatform ,
v1.brand ,
v1.devicestyle ,
v1.ostype ,
count(v1.deviceid) cnt
FROM
v1
GROUP BY
v1.appid ,
v1.appversion ,
v1.appplatform ,
v1.brand ,
v1.devicestyle ,
v1.ostype ;
select * from v4 ;
SELECT
v4.appid ,
v4.appversion ,
v4.appplatform,
v4.brand ,
v4.devicestyle,
v4.ostype ,
ifnull(v3.cnt , 0) / v4.cnt
FROM
v4 left outer join v3
on
v3.appid = v4.appid
and v3.appversion = v4.appversion
and v3.appplatform = v4.appplatform
and v3.brand = v4.brand
and v3.devicestyle = v4.devicestyle
and v3.ostype = v4.ostype

Flume+Kafka+SparkStreaming 最新最全整合
1. 架构
第一步,Flume 和 Kakfa 对接,Flume 抓取日志,写到 Kafka 中
第二部,Spark Streaming 读取 Kafka 中的数据,进行实时分析
本文首先使用 Kakfa 自带的消息处理(脚本)来获取消息,走通 Flume 和 Kafka 的对接
2. 安装 flume,kafka
flume install: http://my.oschina.net/u/192561/blog/692225
kafka install: http://my.oschina.net/u/192561/blog/692357
3.Flume 和 Kafka 整合
3.1 两者整合优势
Flume 更倾向于数据传输本身,Kakfa 是典型的消息中间件用于解耦生产者消费者。
具体架构上,Agent 并没把数据直接发送到 Kafka,在 Kafka 前面有层由 Flume 构成的 forward。这样做有两个原因:
Kafka 的 API 对非 JVM 系的语言支持很不友好,forward 对外提供更加通用的 HTTP 接口。forward 层可以做路由、Kafka topic 和 Kafkapartition key 等逻辑,进一步减少 Agent 端的逻辑。
数据有数据源到 flume 再到 Kafka 时,数据一方面可以同步到 HDFS 做离线计算,另一方面可以做实时计算。本文实时计算采用 SparkStreaming 做测试。
3.2 Flume 和 Kafka 整合安装
1. 下载 Flume 和 Kafka 集成的插件,下载地址:
https://github.com/beyondj2ee/flumeng-kafka- plugin
将 package 目录中的 flumeng-kafka-plugin.jar 拷贝到 Flume 安装目录的 lib 目录下
2. 将 Kakfa 安装目录 libs 目录下的如下 jar 包拷贝到 Flume 安装目录的 lib 目录下
kafka_2.11-0.10.0.0.jar
scala-library-2.11.8.jar
metrics-core-2.2.0.jar
提取插件中的 flume-conf.properties 文件:修改如下:flume 源采用 exec
producer.sources.s.type = exec
producer.sources.s.command=tail -F -n+1 /home/eric/bigdata/kafka-logs/a.log
producer.sources.s.channels = c1
修改 producer 代理的 topic 为 HappyBirthDayToAnYuan
将配置放到 apache-flume-1.6.0-bin/conf/producer.conf 中
完整 producer.conf:
#agentsection
producer.sources= s1
producer.channels= c1
producer.sinks= k1
#配置数据源
producer.sources.s1.type=exec
#配置需要监控的日志输出文件或目录
producer.sources.s1.command=tail -F -n+1 /home/eric/bigdata/kafka-logs/a.log
#配置数据通道
producer.channels.c1.type=memory
producer.channels.c1.capacity=10000
producer.channels.c1.transactionCapacity=100
#配置数据源输出
#设置Kafka接收器,此处最坑,注意版本,此处为Flume 1.6.0的输出槽类型
producer.sinks.k1.type= org.apache.flume.sink.kafka.KafkaSink
#设置Kafka的broker地址和端口号
producer.sinks.k1.brokerList=localhost:9092
#设置Kafka的Topic
producer.sinks.k1.topic=HappyBirthDayToAnYuan
#设置序列化方式
producer.sinks.k1.serializer.class=kafka.serializer.StringEncoder
#将三者级联
producer.sources.s1.channels=c1
producer.sinks.k1.channel=c1
3.3 启动 kafka flume 相关服务
启动 ZK bin/zookeeper-server-start.sh config/zookeeper.properties
启动 Kafka 服务 bin/kafka-server-start.sh config/server.properties
创建主题
bin/kafka-topics.sh --create --zookeeper localhost:2181 --replication-factor 1 --partitions 1 --topic HappyBirthDayToAnYuan
查看主题
bin/kafka-topics.sh --list --zookeeper localhost:2181
查看主题详情
bin/kafka-topics.sh --describe --zookeeper localhost:2181 --topic HappyBirthDayToAnYuan
删除主题
bin/kafka-topics.sh --delete --zookeeper localhost:2181 --topic test
创建消费者
bin/kafka-console-consumer.sh --zookeeper localhost:2181 --topic test --from-beginning
启动 flume
bin/flume-ng agent -n producer -c conf -f conf/producer.conf -Dflume.root.logger=INFO,console
向 flume 发送数据:
echo "yuhai" >> a.log
kafka 消费数据:
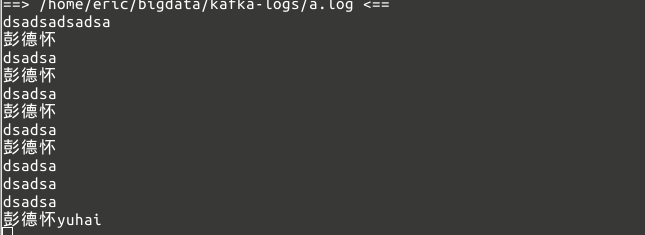
注意:当前文件内容删除,服务器重启,主题需重新创建,但是消费内容有落地文件,当前消费内容不消失.
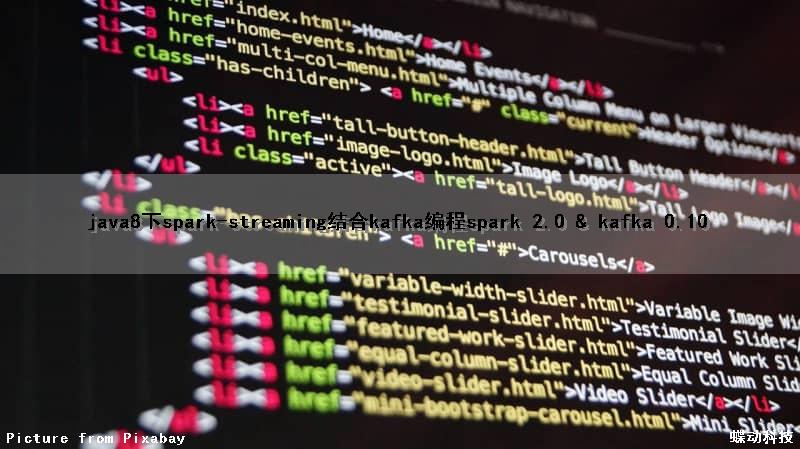
java8下spark-streaming结合kafka编程(spark 2.0 & kafka 0.10
前面有说道spark-streaming的简单demo,也有说到kafka成功跑通的例子,这里就结合二者,也是常用的使用之一。
1.相关组件版本
首先确认版本,因为跟之前的版本有些不一样,所以才有必要记录下,另外仍然没有使用scala,使用java8,spark 2.0.0,kafka 0.10。
2.引入maven包
网上找了一些结合的例子,但是跟我当前版本不一样,所以根本就成功不了,所以探究了下,列出引入包。
<dependency>
<groupId>org.apache.spark</groupId>
<artifactId>spark-streaming-kafka-0-10_2.11</artifactId>
<version>2.0.0</version>
</dependency>
登录后复制
网上能找到的不带kafka版本号的包最新是1.6.3,我试过,已经无法在spark2下成功运行了,所以找到的是对应kafka0.10的版本,注意spark2.0的scala版本已经是2.11,所以包括之前必须后面跟2.11,表示scala版本。
3.SparkSteamingKafka类
需要注意的是引入的包路径是org.apache.spark.streaming.kafka010.xxx,所以这里把import也放进来了。其他直接看注释。
import java.util.Arrays;
import java.util.Collection;
import java.util.HashMap;
import java.util.HashSet;
import java.util.Map;
import org.apache.kafka.clients.consumer.ConsumerRecord;
import org.apache.kafka.common.TopicPartition;
import org.apache.spark.SparkConf;
import org.apache.spark.api.java.JavaSparkContext;
import org.apache.spark.streaming.Durations;
import org.apache.spark.streaming.api.java.JavaInputDStream;
import org.apache.spark.streaming.api.java.JavaPairDStream;
import org.apache.spark.streaming.api.java.JavaStreamingContext;
import org.apache.spark.streaming.kafka010.ConsumerStrategies;
import org.apache.spark.streaming.kafka010.KafkaUtils;
import org.apache.spark.streaming.kafka010.LocationStrategies;
import scala.Tuple2;
public class SparkSteamingKafka {
public static void main(String[] args) throws InterruptedException {
String brokers = "master2:6667";
String topics = "topic1";
SparkConf conf = new SparkConf().setMaster("local[2]").setAppName("streaming word count");
JavaSparkContext sc = new JavaSparkContext(conf);
sc.setLogLevel("WARN");
JavaStreamingContext ssc = new JavaStreamingContext(sc, Durations.seconds(1));
Collection<String> topicsSet = new HashSet<>(Arrays.asList(topics.split(",")));
//kafka相关参数,必要!缺了会报错
Map<String, Object> kafkaParams = new HashMap<>();
kafkaParams.put("metadata.broker.list", brokers) ;
kafkaParams.put("bootstrap.servers", brokers);
kafkaParams.put("group.id", "group1");
kafkaParams.put("key.serializer", "org.apache.kafka.common.serialization.StringSerializer");
kafkaParams.put("key.deserializer", "org.apache.kafka.common.serialization.StringDeserializer");
kafkaParams.put("value.deserializer", "org.apache.kafka.common.serialization.StringDeserializer");
//Topic分区
Map<TopicPartition, Long> offsets = new HashMap<>();
offsets.put(new TopicPartition("topic1", 0), 2L);
//通过KafkaUtils.createDirectStream(...)获得kafka数据,kafka相关参数由kafkaParams指定
JavaInputDStream<ConsumerRecord<Object,Object>> lines = KafkaUtils.createDirectStream(
ssc,
LocationStrategies.PreferConsistent(),
ConsumerStrategies.Subscribe(topicsSet, kafkaParams, offsets)
);
//这里就跟之前的demo一样了,只是需要注意这边的lines里的参数本身是个ConsumerRecord对象
JavaPairDStream<String, Integer> counts =
lines.flatMap(x -> Arrays.asList(x.value().toString().split(" ")).iterator())
.mapToPair(x -> new Tuple2<String, Integer>(x, 1))
.reduceByKey((x, y) -> x + y);
counts.print();
// 可以打印所有信息,看下ConsumerRecord的结构
// lines.foreachRDD(rdd -> {
// rdd.foreach(x -> {
// System.out.println(x);
// });
// });
ssc.start();
ssc.awaitTermination();
ssc.close();
}
}
登录后复制
4.运行测试
这里使用上一篇kafka初探里写的producer类,put数据到kafka服务端,我这是master2节点上部署的kafka,本地测试跑spark2。
立即学习“Java免费学习笔记(深入)”;
UserKafkaProducer producerThread = new UserKafkaProducer(KafkaProperties.topic);
producerThread.start();
登录后复制
再运行3里的SparkSteamingKafka类,可以看到已经成功。
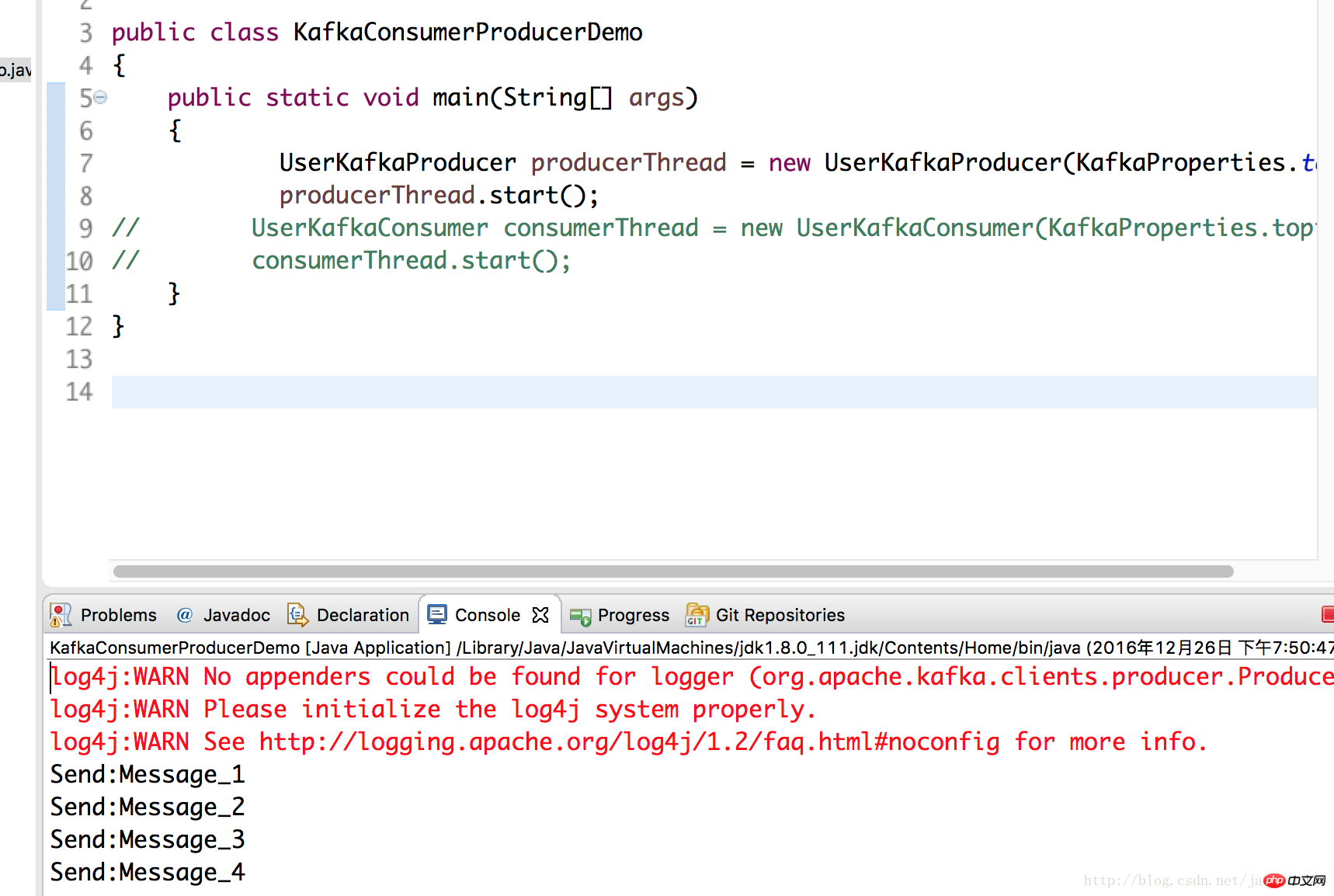
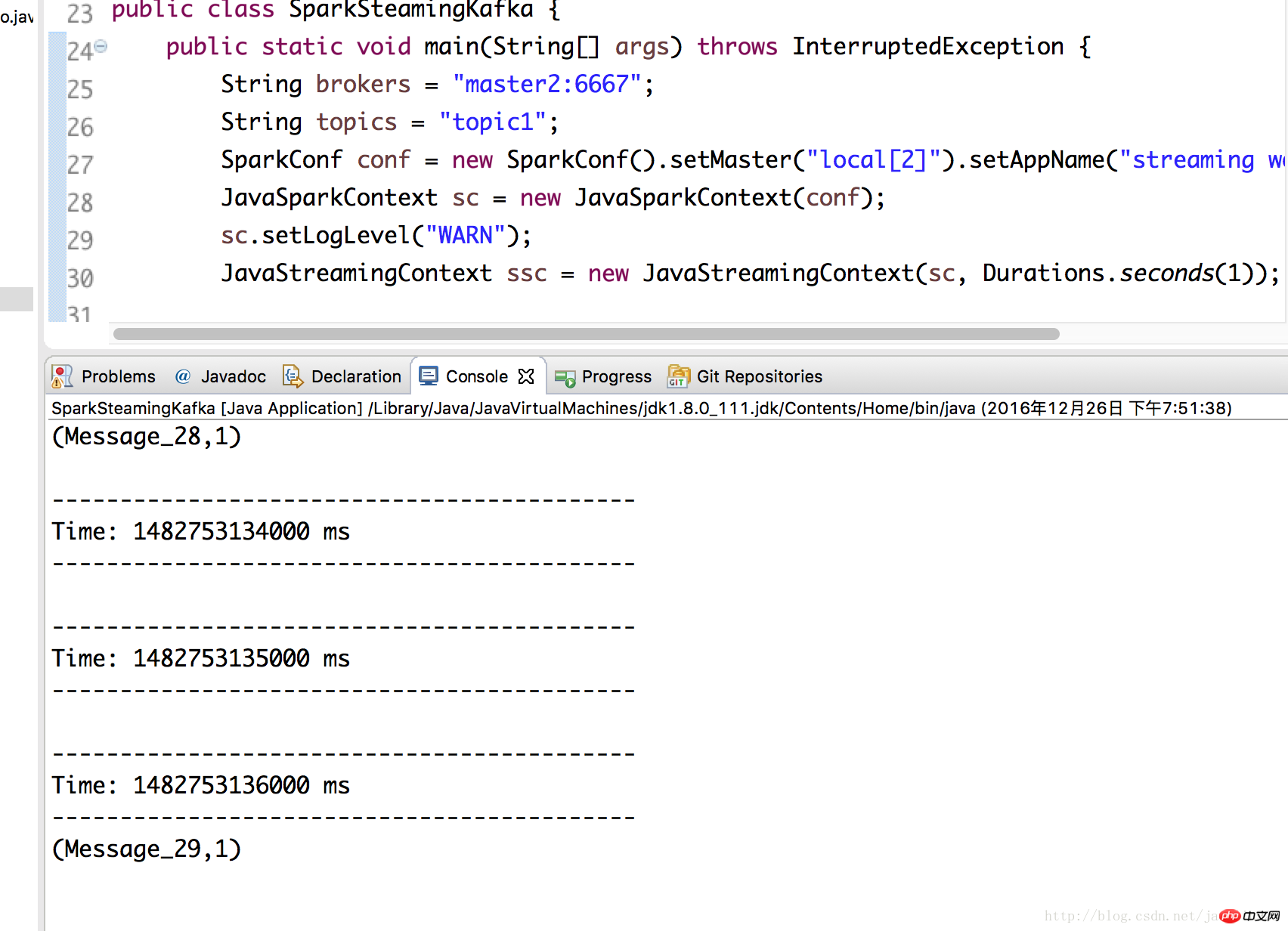
以上就是java8下spark-streaming结合kafka编程(spark 2.0 & kafka 0.10的详细内容,更多请关注php中文网其它相关文章!
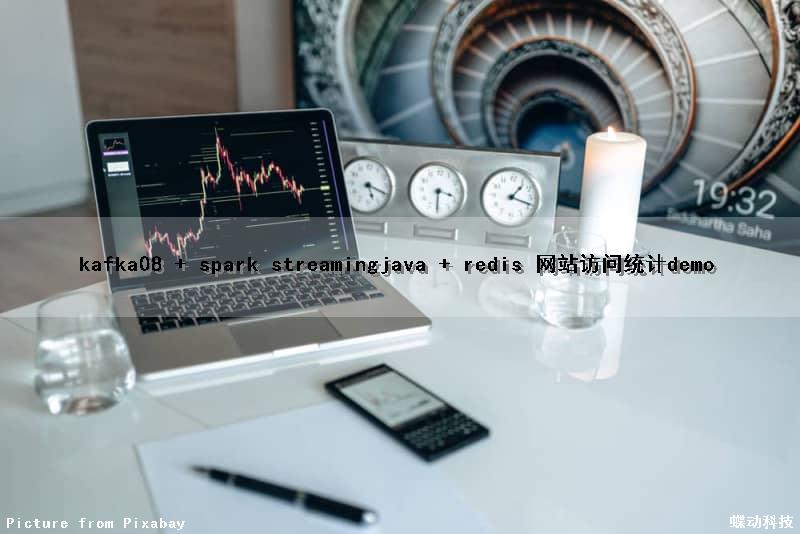
kafka(08) + spark streaming(java) + redis 网站访问统计demo
第一次接触kafka及spark streaming,均使用单机环境。后续改进分布式部署。
本机通过virtual box安装虚拟机,操作系统:ubuntu14,桥接方式,与本机通信,虚拟机地址:192.168.56.102,本机地址:192.168.56.101
虚拟机中部署kafka08,下载地址:
https://www.apache.org/dyn/closer.cgi?path=/kafka/0.8.2.1/kafka_2.11-0.8.2.1.tgz
kafka目录,进入config,server.properties中:
取消host.name的注释:修改为:host.name=192.168.56.102
取消advertised.host.name的注释:修改为:advertised.host.name=192.168.56.102
取消advertised.port的注释:修改为:advertised.port=9092
之后开启zookeeper和kafka进程:
zookeeper : ./kafka/bin/zookeeper-server-start.sh ./kafka/config/zookeeper.properties &
kafka : ./kafka/bin/kafka-server-start.sh ./kafka/config/server.properties &
本地搭建spark streaming 开发环境:
新建java工程,引入spark streaming依赖包:
http://d3kbcqa49mib13.cloudfront.net/spark-2.0.1-bin-hadoop2.7.tgz
引入spark-streaming-kafka依赖包:
spark-streaming-kafka_2.11-1.6.3.jar
编写producer程序:
import java.sql.Time;
import java.util.Properties;
import java.util.Random;
import java.util.concurrent.ExecutionException;
import org.apache.kafka.clients.producer.Callback;
import org.apache.kafka.clients.producer.KafkaProducer;
import org.apache.kafka.clients.producer.Producer;
import org.apache.kafka.clients.producer.ProducerRecord;
import org.apache.kafka.clients.producer.RecordMetadata;
public class ProducerTestSample implements KafkaImpl{
/**
*
*/
private static final long serialVersionUID = 3764795315790960582L;
@Override
public void process() {
Properties props = new Properties();
props.put("bootstrap.servers", "192.168.56.102:9092");
props.put("key.serializer", "org.apache.kafka.common.serialization.StringSerializer");
props.put("value.serializer", "org.apache.kafka.common.serialization.StringSerializer");
Producer<String, String> producer = new KafkaProducer<String, String>(props);
int result = 0;
int multy = 0;
Random random = new Random();
String[] webPages = {"sina","sohu","amazon","google","facebook"};
System.out.println("Producer Test Start Process");
int k = 1;
while(true){
for(int i = 0; i < 100; i++){
result = random.nextInt(4);
multy = random.nextInt(5);
ProducerRecord<String, String> myRecord = new ProducerRecord<String, String>("test2", i+"", webPages[result] + "|" + multy);
producer.send(myRecord,new Callback(){
public void onCompletion(RecordMetadata metadata, Exception e) {
if(e != null) {
e.printStackTrace();
}
}
});
try {
Thread.sleep(1);
} catch (InterruptedException e1) {
e1.printStackTrace();
}
}
if(k==40){
break;
}
k++;
}
producer.close();
}
}
因为结果需要持久化到内存中,需要安装redis数据库。
下载地址:
https://github.com/MSOpenTech/redis/releases
按需下载。
编写consumer,并将结果保存到redis中:
package com.ibm.spark.streaming.test;
import java.util.Collection;
import java.util.Arrays;
import java.util.HashMap;
import java.util.HashSet;
import java.util.Iterator;
import java.util.List;
import java.util.Map;
import java.util.Properties;
import java.util.Set;
import java.util.concurrent.ExecutorService;
import java.util.regex.Pattern;
import org.apache.kafka.clients.consumer.ConsumerRecord;
import org.apache.kafka.clients.consumer.ConsumerRecords;
import org.apache.kafka.clients.consumer.KafkaConsumer;
import org.apache.spark.SparkConf;
import org.apache.spark.api.java.JavaPairRDD;
import org.apache.spark.api.java.function.FlatMapFunction;
import org.apache.spark.api.java.function.Function;
import org.apache.spark.api.java.function.Function2;
import org.apache.spark.api.java.function.PairFunction;
import org.apache.spark.api.java.function.VoidFunction;
import org.apache.spark.streaming.Duration;
import org.apache.spark.streaming.Durations;
import org.apache.spark.streaming.api.java.JavaDStream;
import org.apache.spark.streaming.api.java.JavaInputDStream;
import org.apache.spark.streaming.api.java.JavaPairDStream;
import org.apache.spark.streaming.api.java.JavaPairInputDStream;
import org.apache.spark.streaming.api.java.JavaPairReceiverInputDStream;
import org.apache.spark.streaming.api.java.JavaStreamingContext;
import org.apache.spark.streaming.kafka.KafkaUtils;
import com.google.common.collect.Lists;
import com.ibm.spark.streaming.redis.RedisUtil;
import kafka.serializer.StringDecoder;
import redis.clients.jedis.Jedis;
import scala.Tuple2;
public class ConsumerSample implements KafkaImpl {
/**
*
*/
private static final long serialVersionUID = -7755813648584777971L;
@Override
public void process() {
//Kafka broker version 0.8.2.1
SparkConf sparkConf = new SparkConf().setAppName("JavaDirectKafkaWordCount").setMaster("local[2]");
JavaStreamingContext jssc = new JavaStreamingContext(sparkConf, Durations.seconds(1));
Map<String, Integer> topicMap = new HashMap<>();
String[] topics = {"test2"};
for (String topic: topics) {
topicMap.put(topic, 1);
}
System.out.println("Consumer Start Process");
JavaPairInputDStream<String, String> messages = KafkaUtils.createStream(jssc, "192.168.56.102:2181" ,"0", topicMap);
// Get the lines, split them into words, count the words and print
JavaDStream<String> lines = messages.map(new Function<Tuple2<String, String>, String>() {
/**
*
*/
private static final long serialVersionUID = -5586094486938487203L;
@Override
public String call(Tuple2<String, String> tuple2) {
return tuple2._2;
}
});
JavaDStream<String> words = lines.flatMap(new FlatMapFunction<String, String>() {
/**
*
*/
private static final long serialVersionUID = 5042968941949010821L;
@Override
public Iterator<String> call(String x) {
return Arrays.asList(x).iterator();
}
});
JavaPairDStream<String, Integer> wordCounts = words.mapToPair(
new PairFunction<String, String, Integer>() {
/**
*
*/
private static final long serialVersionUID = -142469460515597388L;
@Override
public Tuple2<String, Integer> call(String s) {
return new Tuple2<>(s, 1);
}
}).reduceByKey(
new Function2<Integer, Integer, Integer>() {
/**
*
*/
private static final long serialVersionUID = 3919864041535570140L;
@Override
public Integer call(Integer i1, Integer i2) {
return i1 + i2;
}
});
wordCounts.foreachRDD(
new VoidFunction<JavaPairRDD<String,Integer>>() {
@Override
public void call(JavaPairRDD<String, Integer> arg0) throws Exception {
// TODO Auto-generated method stub
arg0.foreachPartition(
new VoidFunction<Iterator<Tuple2<String,Integer>>>() {
@Override
public void call(Iterator<Tuple2<String, Integer>> arg0) throws Exception {
Jedis jedis = RedisUtil.getJedis();
while(arg0.hasNext()){
Tuple2<String, Integer> resultT = arg0.next();
System.out.println("(" + resultT._1 + "-----" + resultT._2 + ")");
jedis.lpush("result", "(" + resultT._1 + "-----" + resultT._2 + ")");
}
if(jedis.isConnected())
RedisUtil.returnResource(jedis);
}
});
}
}
);
wordCounts.print();
jssc.start();
try {
jssc.awaitTermination();
} catch (InterruptedException e) {
e.printStackTrace();
}finally{
}
}
}
其中,需要先编写redis的工具类:
package com.ibm.spark.streaming.redis;
import java.io.Serializable;
import redis.clients.jedis.Jedis;
import redis.clients.jedis.JedisPool;
import redis.clients.jedis.JedisPoolConfig;
public class RedisUtil implements Serializable{
/**
*
*/
private static final long serialVersionUID = 264027256914939178L;
//Redis server
private static String redisServer = "127.0.0.1";
//Redis port
private static int port = 6379;
//password
private static String password = "admin";
private static int MAX_ACTIVE = 1024;
private static int MAX_IDLE = 200;
//waittime and timeout time ms
private static int MAX_WAIT = 10000;
private static int TIMEOUT = 10000;
private static boolean TEST_ON_BORROW = true;
private static JedisPool jedisPool = null;
static {
try {
JedisPoolConfig config = new JedisPoolConfig();
config.setMaxActive(MAX_ACTIVE);
config.setMaxIdle(MAX_IDLE);
config.setMaxWait(MAX_WAIT);
config.setTestOnBorrow(TEST_ON_BORROW);
jedisPool = new JedisPool(redisServer, port);
} catch (Exception e) {
e.printStackTrace();
}
}
public synchronized static Jedis getJedis() {
try {
if (jedisPool != null) {
Jedis resource = jedisPool.getResource();
return resource;
} else {
return null;
}
} catch (Exception e) {
e.printStackTrace();
return null;
}
}
/**
* release resource
* @param jedis
*/
public static void returnResource(final Jedis jedis) {
if (jedis != null) {
jedisPool.returnResource(jedis);
}
}
}
之后,编写多线程处理类,调用producer和consumer的process,这样就可以在console中看到输出结果:
-------------------------------------------
Time: 1483597847000 ms
-------------------------------------------
(amazon|2,853)
(sina|3,879)
(sina|1,891)
(amazon|0,880)
(sohu|3,810)
(google|3,920)
(sohu|1,846)
(amazon|4,864)
(google|1,883)
(sina|4,856)
...
-------------------------------------------
Time: 1483597848000 ms
-------------------------------------------
(amazon|2,23)
(sina|3,32)
(sina|1,14)
(amazon|0,15)
(sohu|3,24)
(google|3,25)
(sohu|1,17)
(amazon|4,27)
(google|1,27)
(sina|4,18)
...
-------------------------------------------
Time: 1483597849000 ms
-------------------------------------------
(amazon|2,26)
(sina|3,18)
(sina|1,32)
(amazon|0,31)
(sohu|3,32)
(google|3,29)
同样,在redis客户端中也能查询到相应的结果:
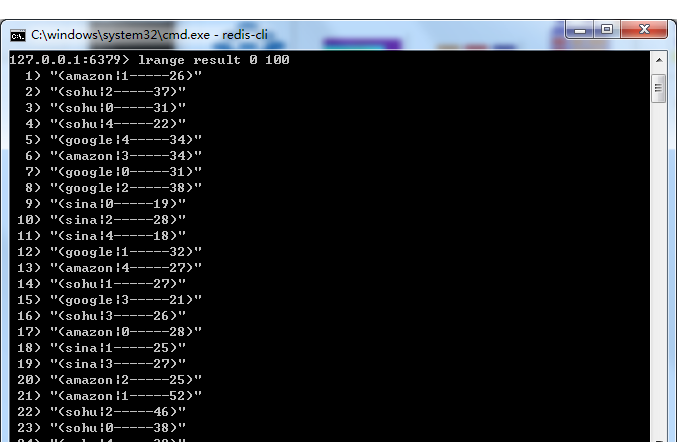
如有问题,敬请讨论。
关于Kafka+Spark Streaming+Redis实时计算整合实践和kafka redis性能的介绍现已完结,谢谢您的耐心阅读,如果想了解更多关于23 友盟项目--sparkstreaming对接kafka、集成redis--从redis中查询月留存率、Flume+Kafka+SparkStreaming 最新最全整合、java8下spark-streaming结合kafka编程(spark 2.0 & kafka 0.10、kafka(08) + spark streaming(java) + redis 网站访问统计demo的相关知识,请在本站寻找。