在本文中,我们将带你了解GuidedProject:PreparingDataForSQLite在这篇文章中,同时我们还将给您一些技巧,以帮助您实现更有效的1:HowGuidedProjectsWor
在本文中,我们将带你了解Guided Project: Preparing Data For SQLite在这篇文章中,同时我们还将给您一些技巧,以帮助您实现更有效的1: How Guided Projects Work(Guided Project: Transforming data with Python )、A cycle was detected in the build path of project Build path Build Path Problem(Eclipse)、All the Apache Streaming Projects: An Exploratory Guide、Android SQLite select * from table where name like%key%using prepared statements。
本文目录一览:- Guided Project: Preparing Data For SQLite
- 1: How Guided Projects Work(Guided Project: Transforming data with Python )
- A cycle was detected in the build path of project Build path Build Path Problem(Eclipse)
- All the Apache Streaming Projects: An Exploratory Guide
- Android SQLite select * from table where name like%key%using prepared statements
Guided Project: Preparing Data For SQLite
1: Introduction To The Data
So far, we''ve learned how to write SQL queries to interact with existing databases. In this guided project, you''ll learn how to clean a CSV dataset and add it to a SQLite database. If you''re new to either our guided projects or Jupyter notebook in general, you can learn morehere. You can find the solutions to this guided project here.
We''ll work with data on Academy Award nominations, which can be downloaded here. The Academy Awards, also known as the Oscars, is an annual awards ceremony hosted to recognize the achievements in the film industry. There are many different awards categories and the members of the academy vote every year to decide which artist or film should get the award. The awards categories have changed over the years, and you can learn more about when categories were added on Wikipedia.
Here are the columns in the dataset,academy_awards.csv
:
Year
- the year of the awards ceremony.Category
- the category of award the nominee was nominated for.Nominee
- the person nominated for the award.Additional Info
- this column contains additional info like:- the movie the nominee participated in.
- the character the nominee played (for acting awards).
Won?
- this column contains eitherYES
orNO
depending on if the nominee won the award.
Read in the dataset into a Dataframe and explore it to become more familiar witht he data. Once you''ve cleaned the dataset, you''ll use a Pandas helper method to export the data into a SQLite database.
Instructions
- Import
pandas
and read the CSV fileacademy_awards.csv
into a Dataframe using theread_csv
method.- When reading the CSV, make sure to set the encoding to
ISO-8859-1
so it can be parsed properly.
- When reading the CSV, make sure to set the encoding to
- Start exploring the data in Pandas and look for data quality issues.
- Use the
head
method to explore the first few rows in the Dataframe. - There are 6 unnamed columns at the end. Use the
value_counts
method to explore if any of them have valid values that we need. - You''ll notice that the
Additional Info
column contains a few different formatting styles. Start brainstorming ways to clean this column up.
- Use the
2: Filtering The Data
The dataset is incredibly messy and you may have noticed many inconsistencies that make it hard to work with. Most columns don''t have consistent formatting, which is incredibly important when we use SQL to query the data later on. Other columns vary in the information they convey based on the type of awards category that row corresponds to.
In the SQL and Databases: Intermediatecourse, we worked with a subset of the same dataset. This subset contained only the nominations from years 2001 to 2010 and only the following awards categories:
Actor -- Leading Role
Actor -- Supporting Role
Actress -- Leading Role
Actress -- Supporting Role
Let''s filter our Dataframe to the same subset so it''s more manageable.
Instructions
-
Before we filter the data, let''s clean up the
Year
column by selecting just the first 4 digits in each value in the column, therefore excluding the value in parentheses:- Use Pandas vectorized string methods to select just the first 4 elements in each string.
- E.g.
df["Year"].str[0:2]
returns a Series containing just the first 2 characters for each element in theYear
column.
- E.g.
- Assign this new Series to the
Year
column to overwrite the original column. - Convert the
Year
column to theint64
data type usingastype
. Make sure to reassign the integer Series object back to theYear
column in the Dataframe or the changes won''t be reflected.
- Use Pandas vectorized string methods to select just the first 4 elements in each string.
-
Use conditional filtering to select only the rows from the Dataframe where the
Year
column is larger than2000
. Assign the new filtered Dataframe tolater_than_2000
. -
Use conditional filtering to select only the rows from
later_than_2000
where theCategory
matches one of the 4 awards we''re interested in.- Create a list of strings named
award_categories
with the following strings:Actor -- Leading Role
Actor -- Supporting Role
Actress -- Leading Role
Actress -- Supporting Role
- Use the
isin
method in the conditional filter to return all rows in a column that match any of the values in a listof strings.- Pass in
award_categories
to theisin
method to return all rows :later_than_2000[later_than_2000["Category"].isin(award_categories)]
- Assign the resulting Dataframe to
nominations
.
- Pass in
- Create a list of strings named
3: Cleaning Up The Won? And Unnamed Columns
Since SQLite uses the integers 0
and 1
to represent Boolean values, convert the Won?
column to reflect this. Also rename the Won?
column to Won
so that it''s consistent with the other column names. Finally, get rid of the 6 extra, unnamed columns, since they contain only null values in our filtered Dataframenominations
.
Instructions
-
Use the Series method
map
to replace allNO
values with0
and allYES
values with1
.- Select the
Won?
column fromnominations
. - Then create a dictionary where each key is a value we want to replace and each value is the corresponding replacement value.
- The following dictionary
replace_dict = { True: 1, False, 0 }
would replace allTrue
values with1
and allFalse
values with0
.
- The following dictionary
- Call the
map
function on the Series object and pass in the dictionary you created. - Finally, reassign the new Series object to the
Won?
column innominations
.
- Select the
-
Create a new column
Won
that contains the values from theWon?
column.- Select the
Won?
column and assign it to theWon
column. Both columns should be in the Dataframe still.
- Select the
-
Use the
drop
method to remove the extraneous columns.- As the required parameter, pass in a list of stringscontaining the following values:
Won?
Unnamed: 5
Unnamed: 6
Unnamed: 7
Unnamed: 8
Unnamed: 9
Unnamed: 10
- Set the
axis
parameter to1
when calling thedrop
method. - Assign the resulting Dataframe to
final_nominations
.
- As the required parameter, pass in a list of stringscontaining the following values:
4: Cleaning Up The Additional Info Column
Now clean up the Additional Info
column, whose values are formatted like so:
MOVIE {''CHARACTER''}
Here are some examples:
Biutiful {''Uxbal''}
-Biutiful
is the movie andUxbal
is the character this nominee played.True Grit {''Rooster Cogburn''}
-True Grit
is the movie andRooster Cogburn
is the character this nominee played.The Social Network {''Mark Zuckerberg''}
-The Social Network
is the movie andMark Zuckerberg
is the character this nominee played.
The values in this column contain the movie name and the character the nominee played. Instead of keeping these values in 1 column, split them up into 2 different columns for easier querying.
Instructions
-
Use vectorized string methods to clean up the
Additional Info
column:- Select the
Additional Info
column and strip the single quote and closing brace ("''}"
) using therstrip
method. Assign the resulting Series object toadditional_info_one
. - Split
additional_info_one
on the string," {''
, using thesplit
method and assign toadditional_info_two
. Each value in this Series object should be a list containing the movie name first then the character name. - Access the first element from each list in
additional_info_two
using vectorized string methods and assign tomovie_names
. Here''s what the code looks like:additional_info_two.str[0]
- Access the second element from each list in
additional_info_two
using vectorized string methods and assign tocharacters
.
- Select the
-
Assign the Series
movie_names
to theMovie
column in thefinal_nominations
Dataframe. -
Assign the Series
characters
to theCharacter
column in thefinal_nominations
Dataframe. -
Use the
head
method to preview the first few rows to make sure the values in theCharacter
andMovie
columns resemble theAdditional Info
column. -
Drop the
Additional Info
column using thedrop
method.
Your Dataframe should look like:
5: Exporting To SQLite
Now that our Dataframe is cleaned up, let''s write these records to a SQL database. We can use the Pandas Dataframe method to_sql
to create a new table in a database we specify. This method has 2 required parameters:
name
- string corresponding to the name of the table we want created. The rows from our Dataframe will be added to this table after it''s created.conn
: the Connection instance representing the database we want to add to.
Behind the scenes, Pandas creates a table and uses the first parameter to name it. Pandas uses the data types of each of the columns in the Dataframe to create a SQLite schema for this table. Since SQLite uses integer values to represent Booleans, it was important to convert the Won
column to the integer values 0
and1
. We also converted the Year
column to theinteger data type, so that this column will have the appropriate type in our table. Here''s the mapping for our columns from the Pandas data type to the SQLite data type:
column | Pandas data type | SQLite data type |
---|---|---|
Year | int64 | integer |
Won | int64 | integer |
Category | object | text |
Nominee | object | text |
Movie | object | text |
Character | object | text |
After creating the table, Pandas creates a largeINSERT
query and runs it to insert the values into the table. We can customize the behavior of the to_sql
method using its parameters. For example, if we wanted to append rows to an existing SQLite table instead of creating a new one, we can set the if_exists
parameter to"append"
. By default, if_exists
is set to"fail"
and no rows will be inserted if we specify a table name that already exists. If we''re inserting a large number of records into SQLite and we want to break up the inserting of records into chunks, we can use the chunksize
parameter to set the number of rows we want inserted each time.
Since we''re creating a database from scratch, we need to create a database file first so we can connect to it and export our data. To create a new database file, we use the sqlite3
library to connect to a file path that doesn''t exist yet. If Python can''t find the file we specified, it will create it for us and treat it as a SQLite database file.
SQLite doesn''t have a special file format and you can use any file extension you''d like when creating a SQLite database. We generally use the.db
extension, which isn''t a file extension that''s generally used for other applications.
Instructions
-
Create the SQLite database
nominations.db
and connect to it.- Import
sqlite3
into the environment. - Use the
sqlite3
methodconnect
to connect to the database filenominations.db
.- Since it doesn''t exist in our current directory, it will be automatically created.
- Assign the returned Connection instance to
conn
.
- Import
-
Use the Dataframe method
to_sql
to exportfinal_nominations
tonominations.db
.- For the first parameter, set the table name to
"nominations"
. - For the second parameter, pass in the Connection instance.
- Set the
index
parameter toFalse
- For the first parameter, set the table name to
6: Verifying In SQL
Let''s now query the database to make sure everything worked as expected.
Instructions
- Import
sqlite3
into the environment. - Create a Connection instance using the
sqlite3
methodconnect
to connect to your database file. - Explore the database to make sure the
nominations
table matches our Dataframe.- Return and print the schema using
pragma table_info()
. The following schema should be returned:Year
:Integer
.Category
:Text
.Nominee
:Text
.Won
:Text
.Movie
:Text
.Character
:Text
.
- Return and print the first 10 rows using the
SELECT
andLIMIT
statements.
- Return and print the schema using
- Once you''re done, use the Connection method
close
to close the connection to the database.
7: Next Steps
In this guided project, you used Pandas to clean a CSV dataset and export it to a SQLite database. As a data scientist, it''s important to learn many tools and how to use them together to accomplish what you need to. As you do more guided projects, you''ll become more familiar with the strengths and weaknesses of each tool. For example, you probably have noticed that data cleaning is much easier in Pandas than in SQL.
- For next steps, explore the rest of our original dataset
academy_awards.csv
and brainstorm how to fix the rest of the dataset:- The awards categories in older ceremonies were different than the ones we have today. What relevant information should we keep from older ceremonies?
- What are all the different formatting styles that the
Additional Info
column contains. Can we use tools like regular expressions to capture these patterns and clean them up?- The nominations for the
Art Direction
category have lengthy values forAdditional Info
. What information is useful and how do we extract it? - Many values in
Additional Info
don''t contain the character name the actor or actress played. Should we toss out character name altogether as we expand our data? What tradeoffs do we make by doing so?
- The nominations for the
- What''s the best way to handle awards ceremonies that included movies from 2 years?
- E.g. see
1927/28 (1st)
in theYear
column.
- E.g. see
Next up is a guided project where we''ll continue down the path we started and explore how to normalize our data into multiple tables using relations.
Back
Introduction to the data
In [ ]:
import pandas as pd df = pd.read_csv("academy_awards.csv", encoding="ISO-8859-1") df
Filtering the data
In [ ]:
df["Year"] = df["Year"].str[0:4] df["Year"] = df["Year"].astype("int64") later_than_2000 = df[df["Year"] > 2000] award_categories = ["Actor -- Leading Role","Actor -- Supporting Role", "Actress -- Leading Role", "Actress -- Supporting Role"] nominations = later_than_2000[later_than_2000["Category"].isin(award_categories)]
Cleaning up the Won? and Unnamed columns
In [ ]:
replacements = { "NO": 0, "YES": 1 } nominations["Won?"] = nominations["Won?"].map(replacements) nominations["Won"] = nominations["Won?"] drop_cols = ["Won?","Unnamed: 5", "Unnamed: 6","Unnamed: 7", "Unnamed: 8", "Unnamed: 9", "Unnamed: 10"] final_nominations = nominations.drop(drop_cols, axis=1)
Cleaning up the Additional Info column
In [ ]:
additional_info_one = final_nominations["Additional Info"].str.rstrip("''}") additional_info_two = additional_info_one.str.split(" {''") movie_names = additional_info_two.str[0] characters = additional_info_two.str[1] final_nominations["Movie"] = movie_names final_nominations["Character"] = characters final_nominations = final_nominations.drop("Additional Info", axis=1) final_nominations
Exporting to SQLite
In [ ]:
import sqlite3 conn = sqlite3.connect("nominations.db") final_nominations.to_sql("nominations", conn, index=False)
Verifying in SQL
In [ ]:
query_one = "pragma table_info(nominations);" query_two = "select * from nominations limit 10;" print(conn.execute(query_one).fetchall()) print(conn.execute(query_two).fetchall()) conn.close()
1: How Guided Projects Work(Guided Project: Transforming data with Python )
Welcome to the first Dataquest guided project! Guided projects are a way to help you synthesize concepts learned during the Dataquest missions, and start building a portfolio. Guided projects go above and beyond regular projects by providing an in-browser coding experience, along with help and hints. Guided projects bridge the gap between learning using the Dataquest missions, and applying the knowledge on your own computer.
Guided projects help you develop key skills that you''ll need to perform data science work in the "real world". Doing well on these projects is slightly different from doing well in the missions, where there is a "right" answer. In the guided projects, you''ll need to think up and create solutions on your own (although we''ll be there to help along the way).
The guided project interface is structured much like an IDE on your local machine would be. This area contains text and instructions. You can advance between steps in the project whenever you want -- since there''s no "right" answer for any screen, the text is mainly for you to use as a reference and guide as you build the project. To the right is a file browser interface, where you can view, create, and edit files. Under the file browser is a terminal window, where you can run shell commands.
Note: Only files stored in the project folder, in this case /home/dq/scripts
, will be saved! If you make changes to files elsewhere, they won''t be saved.
As you go through this project, Google,StackOverflow, and the documentation for various packages will help you along the way. All data scientists make extensive use of these and other resources as they write code, and so should you.
We''d love to hear your feedback as you go through this project, and we hope it''s a great experience!
Instructions
For now, just hit "Next" to get started with the project!
###################################################
2: The Dataset
In this project, you''ll be working with a dataset of submissions to Hacker News from 2006 to 2015. Hacker News is a site where users can submit articles from across the internet (usually about technology and startups), and others can "upvote" the articles, signifying that they like them. The more upvotes a submission gets, the more popular it was in the community. Popular articles get to the "front page" of Hacker News, where they''re more likely to be seen by others.
The dataset you''ll be using was compiled by Arnaud Drizard using the Hacker News API, and can be found here. We''ve sampled 10000
rows from the data randomly, and removed all extraneous columns. Our dataset only has four columns:
submission_time
-- when the story was submitted.upvotes
-- number of upvotes the submission got.url
-- the base domain of the submission.headline
-- the headline of the submission. Users can edit this, and it doesn''t have to match the headline of the original article.
You''ll be writing scripts to answer some main questions:
- What words appear most often in the headlines?
- What domains were submitted most often to Hacker News?
- At what times are the most articles submitted?
You''ll be answering these questions by writing command line scripts, instead of using IPython notebook. IPython notebooks are great for quick data visualization and exploration, but Python scripts are the way to put anything we learn into production. Let''s say you want to make a website to help people write headlines that get as many upvotes as possible, and submit articles at the right time. To do this, you''ll need scripts
####################################################
3: Reading The Data
There should be a file called read.py
already open. You can run this from the command line by being in the same folder, and typing python read.py
. Of course, there''s nothing in the file right now. You might recall from the last mission that you can put this into a file to run it from the command line:
if __name__ == "__main__":
print("Welcome to a Python script")
This will print Welcome to a Python script
on the command line if you put it into a file and run it.
We can also add functions into a file by writing them like normal:
def load_data():
pass
if __name__ == "__main__":
# This will call load_data if you
run the script from the command
line.
load_data()
Function definitions should come before the if __name__ == "__main__"
line. These functions can be imported from other files.
We''ll be adding some code to the read.py
file that will help us load in the dataset and do some initial processing. We''ll then be able to import the code to read in the dataset from other scripts we develop.
Instructions
- In the
read.py
file, read thehn_stories.csv
file into a Pandas Dataframe. - There is no header row in the data, so the columns don''t have names. See this stackoverflow thread for how to add column names. Add the column names from the last screen (
submission_time
,upvotes
,url
, andheadline
) to the Dataframe. - Create a function called
load_data
that takes no inputs, but contains the code to read in and process the dataset.load_data
should return a Pandas Dataframe with the column names set correctly.
As you work on these steps, you should be running your script on the command line every so often and verifying that things are working. You can run read.py
from the command line by calling python read.py
. The first verification is to make sure that you don''t see any errors. The second one is to call print
at key points in your code, and make sure that the output looks like what you expect. You might want to do this after each step above. This is a good general rule of thumb to follow when writing new code
############################################
4: Which Words Appear In The Headlines Often?
We now want to figure out which words appear most often in the headlines. We''ll be developing another script, called count.py
to accomplish this. We''ll need to import our load_data
function from read.py
into count.py
so we can use it.
You''ll recall that if you have a folder with two files, read.py
and count.py
, you can use the function load_data
in read.py
fromcount.py
by writing the following code incount.py
:
import read
df = read.load_data()
Instructions
Writing the script for this will require a series of steps:
- Make a file called
count.py
, using the file browser, or the command line. - Import
load_data
fromread.py
, and call the function to read in the dataset. - The order in which you do the below two steps is up to you, but it''s suggested to first combine all the headlines (you can use afor loop for this, among other methods), and then split everything into words.
- Combine all of the headlines together into one long string. You''ll want to leave a space between each headline when you combine them. Here''s a good reference on joining strings.
- Figure out how to split the long string into words. Each headline is a string, such as
Anticlimax As Motivation Killer
. Combining that withSwype acquired by Nuance for 100 million
would look likeAnticlimax As Motivation Killer Swype acquired by Nuance for 100 million
. Adding more headlines would make a longer string. You''ll need to figure out a way to split the long string, so you end up with a list of words. The documentation for str might help here.
- You might want to think about lowercasing each word, so
Hello
andhello
aren''t treated as different words when you do a count. - Find a way to count up how many times each word occurs in the list. The Counter class might help you.
- Add code to print the
100
words that occur the most in your data
A cycle was detected in the build path of project Build path Build Path Problem(Eclipse)
解决:window--preferences--java--Compiler--Building--Build path problems--circular dependencies 由 error 改成 warning 并应用,问题解决。
All the Apache Streaming Projects: An Exploratory Guide
The speed at which data is generated, consumed, processed, and analyzed is increasing at an unbelievably rapid pace. Social media, the Internet of Things, ad tech, and gaming verticals are struggling to deal with the disproportionate size of data sets. These industries demand data processing and analysis in near real-time. Traditional big data-styled frameworks such as Apache Hadoop is not well-suited for these use cases.
As a result, multiple open source projects have been started in the last few years to deal with the streaming data. All were designed to process a never-ending sequence of records originating from more than one source. From Kafka to Beam, there are over a dozen Apache projects in various stages of completion.
With a high overlap, the current Apache streaming projects address similar scenarios. Users often find it confusing to choose the right open source stack for implementing a real-time stream processing solution. This article attempts to help customers navigate the complex maze of Apache streaming projects by calling out the key differentiators for each. We will discuss the use cases and key scenarios addressed by Apache Kafka, Apache Storm, Apache Spark, Apache Samza, Apache Beam and related projects.
Apache Flume
Apache Flume is one of the oldest Apache projects designed to collect, aggregate, and move large data sets such as web server logs to a centralized location. It belongs to the data collection and single-event processing family of stream processing solutions. Flume is based on an agent-driven architecture in which the events generated by clients are streamed directly to Apache Hive, HBase or other data stores.
Flume’s configuration includes a source, channel, and sink. The source can be anything from a Syslog to the Twitter stream to an Avro endpoint. The channel defines how the stream is delivered to the destination. The valid options include Memory, JDBC, Kafka, File among others. The sink determines the destination where the stream gets delivered. Flume supports many sinks such as HDFS, Hive, HBase, ElasticSearch, Kafka and others.
Apache Flume is ideal for scenarios where the client infrastructure supports installing agents. The most popular use case is to stream logs from multiple sources to a central, persistent data store for further processing analysis.
Sample Use Case: Streaming logs from multiple sources capable of running JVM.
Apache Spark
Apache Spark is the hottest technology in the big data ecosystem. It gained the attention of data scientists and developers for its fast, in-memory processing capabilities combined with expressive development APIs. Spark was originally developed at the University of California, Berkeley’s AMPLab, which was later donated to the Apache Software Foundation.
Apache Spark provides developers with an API that’s centered around a data structure called the resilient distributed dataset (RDD), which is a read-only multiset of data items distributed over a cluster of machines, which is fault-tolerant. Spark is designed to overcome the limitations of MapReduce where RDDs function as a working set for distributed programs taking advantage of distributed shared memory. Spark claims to be 100 times faster than Hadoop MapReduce in memory, or 10 times faster when run on disk.
Spark is written in Scala but supports multiple programming languages. It comes with adapters for working with data stored in diverse sources, including HDFS files, Cassandra, HBase, and Amazon S3.
Spark Streaming is an essential component for building fault-tolerant streaming applications. It enables developers to build streaming applications through Sparks’ high-level API. Since it runs on Spark, Spark Streaming lets developers reuse the same code for batch processing, join streams against historical data, or run ad-hoc queries on stream state. It can be used to build powerful interactive applications beyond traditional analytics. Spark Streaming operates in micro-batching mode where the batch size is much smaller to conventional batch processing.
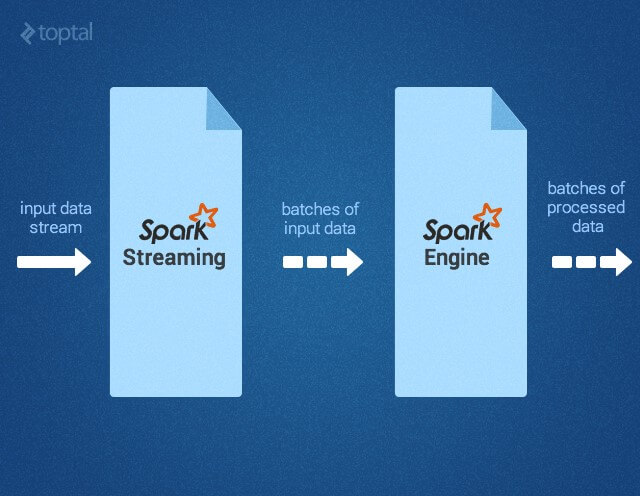
Source: Toptal
Though not a strict requirement, Spark can be run on existing Hadoop and Mesos clusters. It provides a shell for exploring data interactively.
Apache Spark, when combined with Apache Kafka, delivers a powerful stream processing environment.
Sample Use Case: Processing social media feeds in real-time for performing sentiment analysis.
Apache Storm
Apache Storm was originally developed by Nathan Marz at BackType, a company that was acquired by Twitter. After the acquisition, Twitter open sourced Storm before donating it to Apache. Trusted by companies such as Flipboard, Yahoo!, and Twitter, it has emerged as the standard for developing distributed, real-time, data processing platforms.
Storm is often referred as the Hadoop for real-time processing. According to the official documentation, “Storm makes it easy to reliably process unbounded streams of data, doing for realtime processing what Hadoop did for batch processing.”
Apache Storm is primarily designed for scalability and fault-tolerance. It guarantees that every tuple will be processed at least once. Though it is written in Clojure, applications can be written in any programming language that can read and write to standard input and output streams. Storm is designed to support connecting input streams, called as “spouts” and “bolts,” which are processing and output modules. A collection of spouts and bolts form directed acyclic graph (DAG), which is called as a topology. Based on the pre-defined configuration, topologies run on clusters with the scheduler distributing the work across nodes that are part of the cluster.
Storm topologies are often compared to Hadoop MapReduce jobs. But unlike Hadoop jobs, topologies run continuously till they are terminated. Within a topology, spouts acquire the data which will go through a series of bolts. Each bolt is responsible for transforming or processing the data. Some bolts may write the data into persistent databases or files while others may call third party APIs to transform data.
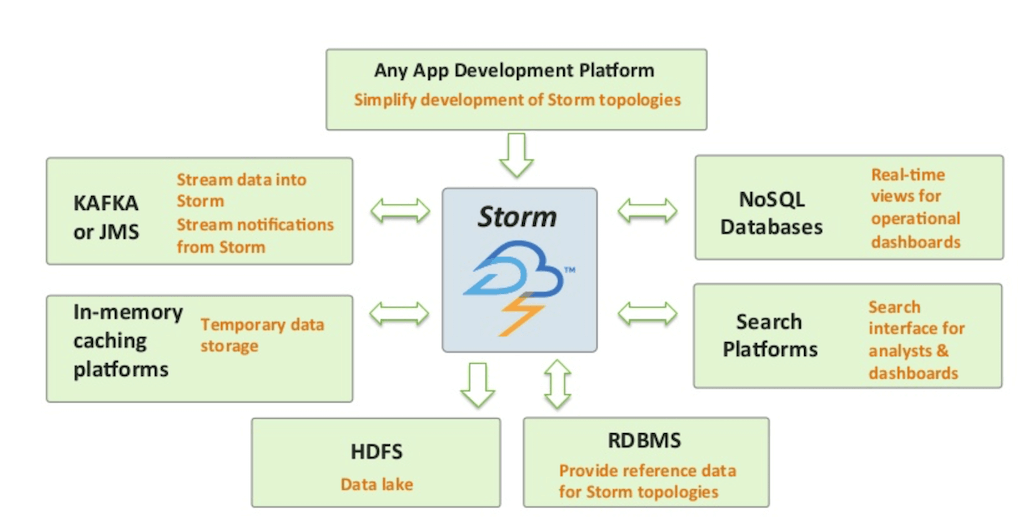
Source: Hortonworks
Thanks to the open source ecosystem, there is a rich collection of spouts for popular data sources, which were created by the community. Through the concepts of adapters, Storm can interoperate with HDFS file systems to participate in Hadoop jobs.
Storm is commonly used in combination with other data ingestion and processing components such as Apache Kafka and Apache Spark. It delivers a reliable, scalable, fault-tolerant distributed computing framework.
Sample Use Case: Transform and process social media / IoT sensor streams in real-time.
Apache NiFi
When compared to other streaming solutions, Apache NiFi is a relatively new project that got graduated to become an Apache Top-Level project in July 2015. It is based on Enterprise Integration Patterns (EIP) where the data flows through multiple stages and transformations before reaching the destination.
Apache NiFi comes with a highly intuitive graphical interface that makes it easy to design data flow and transformations. Business analysts and decision makers can use the tool to define the data flow. It supports a variety of input sources that include both static and streaming data sets. Data that’s acquired from sources such as file system, social media streams, Kafka, FTP, HTTP, JMS, can flow to a variety of destinations including ElasticSearch, Amazon S3, AWS Lambda, Splunk, Solr, SQL, and NoSQL databases. Transformations can be introduced into the path of the data flow.
The emerging area of industrial IoT demands a robust, reliable, and secure data flow engine. Apache NiFi has the potential to become the most preferred orchestration engine for processing sensor data in IoT implementations. It offers the simplicity of Node-Red with the power of Big Data. Inbuilt support for Kafka, JMS, and other channels makes it an ideal choice for enterprise IoT solutions.
One of the classic scenarios that Apache NiFi addresses is the creation of hot path and cold path analytics. The data sets generated by IoT devices and sensor contain certain data points that need to be analyzed in real-time while a subset of the data is stored for batch processing. Such data sets are typically streamed via high-velocity engines such as Apache Kafka, Amazon Kinesis, and Azure Event Hubs. Apache NiFi can be used to define two separate paths for the same data sets responsible for near real-time processing (hot path) and batch processing (cold path).
Sample Use Case: An interactive rules engine to define the flow of IoT sensor data.
Apache Apex
DataTorrent, a Silicon Valley-based company, donated one of its real-time streaming commercial products to Apache Foundation, which is now called Apache Apex. It’s one of the youngest projects at Apache that got graduated from the incubator to become a Top-Level Project. Apache Apex is positioned as an alternative to Apache Storm and Apache Spark for real-time stream processing. It’s claimed to be at least 10 to 100 times faster than Spark.
When compared to Apache Spark, Apex comes with enterprise features such as event processing, guaranteed order of event delivery, and fault-tolerance at the core platform level. Unlike Spark, which needs strong Scala skills, Apex can be used by exiting Java developers. It is designed to run well within the existing Hadoop ecosystem, using YARN for scaling up or down while using HDFS for fault tolerance.
Apache Apex is positioned as industry’s only open-source enterprise-grade engine capable of handling batch data as well as streaming data needs. It is a data-in-motion platform that allows for a unification of processing of real-time streams of unbounded data (streaming job), or bounded data in conventional files (batch job). Organizations can build applications to suit their business logic, and extend the applications across batch processing as well as streaming jobs. Apache Apex architecture can handle reading from and writing to message buses, file systems, databases or any other sources. As long as these sources have client code that can be run within a JVM, the integration works seamlessly.
Apex comes with a library of operators called Malhar, which are pre-built operators for data sources and destinations such as message buses, file systems, and databases. These operators enable developers to build quickly business logic that deals with a variety of data sources. The overall goal of Apex is to reduce the complexity of Big Data projects in enterprises.
Sample Use Case: Applications running on a fault-tolerant infrastructure that are required to process heterogeneous data sets in real-time as well as in batch-mode.
Apache Kafka Streams
Kafka Streams is just a library built on top of the popular data ingestion platform, Apache Kafka. The source code is available as a part of Kafka project. It’s contributed by Confluent, a startup that’s founded by the original developers of Kafka project at LinkedIn.
During the recent past, Apache Kafka emerged as the most popular real-time, large-scale messaging system. It has quickly become the core infrastructure building block for contemporary data platforms. It is used across a wide range of industries by thousands of companies, including Netflix, Cisco, PayPal, and Twitter. Kafka is also exposed as a managed service by the public cloud providers offering hosted Big Data and analytics platforms.
Kafka Streams is a library for building streaming applications, specifically those applications that dealing with transforming input Kafka topics into output Kafka topics. It is not designed for large analytics but for microservices that deliver efficient and compact stream processing. What this means is that the Kafka Streams library is designed to be integrated into the core business logic of an application rather than being a part of a batch analytics job.
Kafka Streams relieve users from setting up, configuring, and managing complex Spark clusters solely deployed for stream processing. It simplifies stream processing to make it accessible as a stand-alone application programming model for asynchronous services. Developers can embed Kafka Streams functionality without the need for a stream processing cluster. The architecture will have Apache Kafka and an application without an external dependency. Kafka Streams deliver a processing model that is fully integrated with the core abstractions Kafka provides to reduce the total number of moving pieces in a stream architecture. It is not a part of MapReduce code that’s typically written to deal with batch processing.
While discussing Kafka Streams, it’s also important to touch upon Kafka Connect, which is a framework for reliably connecting Kafka with external systems such as databases, key-value stores, search indexes, and file systems.
The best thing about Kafka Streams is that it can be packaged as a container that can be on Docker. DevOps teams can also use Ansible, Puppet, Chef, Salt, or even shell script to deploy and manage the application. Once packaged as a container, it can be integrated with orchestration engines such as Docker Swarm, Kubernetes, DC/OS, Yarn, and others.
Sample Use Case: Microservices and stand-alone applications that need embedded stream processing capabilities without the dependency on complex clusters.
Apache Samza
Apache Samza was developed at LinkedIn to avoid the large turn-around times involved in Hadoop’s batch processing. It is built on top of Apache Kafka, a low-latency distributed messaging system. Samza was built to provide a lightweight framework for continuous data processing.
The combination of Kafka and Samza is analogous to HDFS and MapReduce. If HDFS acts as the input for MapReduce jobs, Kafka ingests data processed by Samza. Samza can continuously compute results as and when the data arrives delivering sub-second response times.
After getting the input from streams, Samza executes a job, which is the code that consumes and processes a set of input streams. Jobs may be written in Java, Scala or other languages that support JVM. For scalability, jobs are further broken down into smaller execution units called tasks, which is the unit of parallelism, just as the partition is to the stream. Each task consumes data delivered by one of the partitions. A task processes messages from each of its input partitions sequentially, in the order of message offset. There is no defined ordering across partitions, allowing each task to operate independently.
Samza groups multiple tasks that are executed inside one or more containers, which are isolated OS processes running a JVM that is responsible for executing a set of tasks for a single job. Containers are single threaded which are responsible for managing the lifecycle of tasks.
The key difference between Samza and other streaming technologies lies in its stateful streaming processing capability. Samza tasks have dedicated key/value store co-located on the same machine as the task. This architecture delivers better read/write performance than any other streaming processing software.
Since Samza evolved from extensive usage of Kafka at LinkedIn, they have a great compatibility. It becomes a natural choice in architectures where Kafka is used for ingestion.
Apache Samza and Kafka Streams address the same problem with the later being an embeddable library than a full-fledged software.
Sample Use Case: Optimized stream processing for applications utilizing Kafka for ingestion.
Apache Flink
Apache Flink was originally developed as “Stratosphere: Information Management on the Cloud” in 2010 at Germany as a collaboration of Technical University Berlin, Humboldt-Universität zu Berlin, and Hasso-Plattner-Institut Potsdam. After its submission to Apache Software Foundation, it became a Top-Level Project in December 2014. At first, The concepts and use cases of Apache Flink looks similar to Apache Spark. It aims to be a single platform for running batch, streaming, interactive, graph processing and machine learning applications. But there are differences in the implementation between Spark and Flink.
Spark Streaming is designed to deal with mini batches which can deliver near real-time capabilities. Apache Flink delivers real-time processing due to the fine-grained event level processing architecture.
Flink brings a few unique capabilities to stream processing. It provides exactly-once guarantees to state updates freeing the developers from the burden of dealing with duplicates. It has a high-throughput engine which can buffer events before they sent over the distributed network. Flink provides a powerful streaming programming model with flexible windowing schemes.
Flink is built to be both, a DataStream API for stream analytics and a DataSet API for batch analytics on top of the underlying stream processing engine.
Apache Flink supports programs written in Java or Scala, which get automatically compiled and optimized into data flow programs. Flink does not have its data storage system. The input data can come from a distributed storage system like HDFS or HBase. For data stream processing, Flink can consume data from message queues such as Kafka.
Sample Use Case: Detection and prevention of fraudulent credit card transactions in real-time.
Apache Beam
Apache Beam is the latest addition to the growing list of streaming projects at the Apache Software Foundation. The name of this project signifies the design, which is a combination of Batch and Stream processing models. It is based on a unified model for defining and executing data-parallel processing pipelines that come with a set of language-specific SDKs for constructing pipelines and runtime-specific Runners for executing them.
Google, along with data Artisans, Cloudera, and PayPal donated the SDK of its Big Data services, Cloud Dataflow to ASF, which has become the foundation of Apache Beam. It evolved from a variety of internal Google projects such as MapReduce, FlumeJava, and Millwheel. The concept of Pipeline Runners in Beam translates data processing pipelines into an API that’s compatible with multiple distributed processing backends. A pipeline is a chain of processes that work on a dataset. Each Beam Program will have a runner for the back-end depending on where the pipeline is executed. The platform currently supports runners including Google Cloud Dataflow, Apache Flink, and Apache Spark. Other runners such as Storm and MapReduce are in works.
What problem does Beam solve? When MapReduce jobs get migrated from Hadoop to Spark or Flink, a lot of refactoring is required. Dataflow attempts to be an abstraction layer between the code and execution runtime. When the code is implemented in Dataflow SDK, it will run on multiple backends such as Flink and Spark. Beam supports Java and Python with other language bindings in the works. It aims at bringing multiple languages, frameworks, and SDKs into one unified programming model.
Sample Use Case: Applications that depend on multiple frameworks including Flink and Spark.
Apache Ignite
Apache Ignite is an in-memory layer built on top of a distributed in-memory computing platform. It is optimized to process large data sets in real time. The in-memory architecture makes it much faster than what is possible with traditional disk-based or flash-based technologies.
The project was originally developed by GridGain Systems which donated it to ASF in 2014. In September 2015, Ignite graduated from incubation to become a TLP.
Though both Spark and Ignite rely on distributed in-memory processing architecture, there is subtle difference between the two. Spark is primarily designed for interactive analytics and machine learning applications, while Ignite is meant to deliver programmatic real-time analytics, machine-to-machine communication, and high-performance transactional processing.
Ignite has the potential to become the preferred solution for transaction processing systems such as stock trading, fraud detection, real-time modeling and analysis. It works equally well with scale-out architecture running on commodity hardware or with vertical scaling on high-end workstations and servers.
Ignite streaming feature allows processing continuous never-ending streams of data in scalable and fault-tolerant fashion. The rates at which data can be injected into Ignite can be very high and easily exceed millions of events per second on a moderately sized cluster.
Sample Use Case: Applications that heavily rely on programmatic real-time analytics, machine-to-machine communication, and high-performance transactional processing.
Android SQLite select * from table where name like%key%using prepared statements
我想使用预备的语句来防止在sql sql数据库上的sql注入.但是,当查询包含Like并且与Where name =?一起工作时,rawquery会崩溃?
有没有办法在Android sqlite数据库中使用类似和准备好的语句?
这是查询:
sqlQuery = "SELECT * FROM " + TABLE_CALLS + " where " + CALLER_NAME + " like ? COLLATE NOCASE or " + CALLER_NBR + " like ? or " + CALLER_EXT + " like ?" + " or " + IS_OUTGOING + " like ? COLLATE NOCASE or " + TYPE + " like ? COLLATE NOCASE"; Cursor cursor = database.rawQuery(sqlQuery,new String[]{"%" + criterion + "%","%" + criterion + "%","%" + criterion + "%"});
它会使绑定或列索引超出范围
谢谢.
解决方法
if (name.length() != 0) { name = "%" + name + "%"; } if (email.length() != 0) { email = "%" + email + "%"; } if (Phone.length() != 0) { Phone = "%" + Phone + "%"; } String selectQuery = " select * from tbl_Customer where Customer_Name like '" + name + "' or Customer_Email like '" + email + "' or Customer_Phone like '" + Phone + "' ORDER BY Customer_Id DESC"; Cursor cursor = mDb.rawQuery(selectQuery,null);`
今天的关于Guided Project: Preparing Data For SQLite的分享已经结束,谢谢您的关注,如果想了解更多关于1: How Guided Projects Work(Guided Project: Transforming data with Python )、A cycle was detected in the build path of project Build path Build Path Problem(Eclipse)、All the Apache Streaming Projects: An Exploratory Guide、Android SQLite select * from table where name like%key%using prepared statements的相关知识,请在本站进行查询。
本文标签: